Statistical Bootstrapping Method Using a Bias-Corrected Acceleration Approach : Take the Uncertainty out of Drug Development
Well-reasoned and properly conducted statistical analyses can be essential to successful drug development, particularly in the context of manufacturing scale-up, process and component changes, and in comparisons with a reference product. Similarity tests between dissolution profiles steer the development path forward based on the quality and interpretation of the results. When the wrong statistical analysis method is applied, it can lead a development program completely off its rails. But does the choice of statistical analysis method for highly variable dissolution data have to lead to uncertainty?
The Challenge
Dissolution datasets are commonly used to compare manufactured drug products from different lots or from different manufacturers. Because in vitro dissolution analytical methods can serve as a proxy for in vivo product disintegration, dissolution, active ingredient release, and availability for uptake into the systemic circulation, statistical comparisons between lots or drug products’ dissolution data are common. In the context of drug development and regulatory approvals, comparisons are most often used to show similarities or differences between a reference listed drug and a newly manufactured generic version (e.g., as in an ANDA); a different formulation of a solid oral dosage form (e.g., as in a 505(b)(2) NDA); and comparisons between lots of the same drug product manufactured before and after scale increases, or after component, manufacturing scale, and process changes that may occur (or be considered) before and after product approval.
Premier Consulting is deeply familiar with the challenges of performing FDA-acceptable calculations for the similarity factor (f2) when highly variable dissolution data is present. Our experience with more than 200 FDA approvals has driven our team to develop a statistical methodology and associated programming that performs consistent analysis to calculate the similarity factor (f2) using the bias-corrected and accelerated (BCA) bootstrapping method approach.
Statistical methodologies are complex when dissolution datasets are highly variable. This variability can cause a great deal of uncertainty, which can have a significant impact on the clarity of the statistical output and the regulatory conclusions that can be drawn from it. Ultimately, the success of a product-development program and a drug’s FDA approval—particularly for 505(b)(2) and ANDA programs—can rest on the statistical approach used and whether it is reliable or not.
The Road to the BCA Method
The Premier Consulting team meets with the FDA over 75 times per year, which helps our regulatory scientists understand the FDA’s current thinking on the preferred technical and strategic approaches to drug development. From our many interactions with the FDA, we have confirmed that the FDA prefers the bootstrap method with BCA to evaluate the similarity factor, f2, for highly variable dissolution data.
One of the regulatory requirements is to estimate the similarity factor (f2) to determine the similarity between the dissolution profiles of the test (post-change) and reference (pre-change) products (12 units each). The f2 calculation uses mean dissolution values. However, when the variability of dissolution data is high (more than 20 percent (%) coefficient of variation (CV), at earlier time points (e.g., 15 minutes) or more than 10% at other time points) other statistical models should be considered.
Q&A with Premier Consulting Biopharmaceutical Scientists
In order to best convey the background and substance of this complex topic, we have conducted this discussion as a question and answer with Premier Consulting Strategic Advisor Dr. Ruth Stevens.
How did you become interested in this topic?
Part of the journey is based on previous discussions and research on Scientific and Regulatory Standards for Assessing Product Performance Using the Similarity Factor, f2, and on a scientific roundtable at American Association of Pharmaceutical Scientists Annual Meeting. In 2013, the FDA invited me to write a commentary article from the scientific roundtable (here).
Why is the bootstrap method with BCA important?
For NDAs, many applicants don’t fully understand which test is acceptable to the FDA for highly variable dissolution data. There is no clear published guidance for specific tests that the FDA recommends an applicant to use, so Premier Consulting has developed expertise in this matter.
This opens up conversations with the FDA on which statistical approach, given the particular circumstances of a specific drug product, is most relevant for approval.
When is this method most important to be used?
If the assumptions of the statistical tests are met there will be very little difference in the results, thus various methods of statistical analysis can be used. However, the bootstrap method with BCA doesn’t need to assume the data is normally distributed, giving it a wider versatility in calculation to produce reliable results.
What are the top two advantages associated with the bootstrap method with BCA?
The top two advantages are: 1) it enables a reliable result even when highly variable dissolution data sets are being compared and analyzed. 2) FDA has concluded that this methodology can be very useful for regulatory decision-making in this context. The SAS code that Premier Consulting developed for the bootstrap method is used to calculate the 90% CIs using bias-corrected and accelerated (BCA) approaches.
What is the basic approach to this bootstrap method?
Often with highly variable dissolution data, a few tablets are considered “outliers” which would have more impact on the mean dissolution time point result than others (an outlier is commonly defined as a point that falls more than 1.5 times the interquartile range above the third quartile or below the first quartile). The BCA bootstrap method is used to gain a more reliable result that limits the impact of any outliers. If the 90% CI lower limit of BCA bootstrapped f2 is equal to or greater than 50, the similarity between the test and reference products is demonstrated, even considering the high variability and outliers.
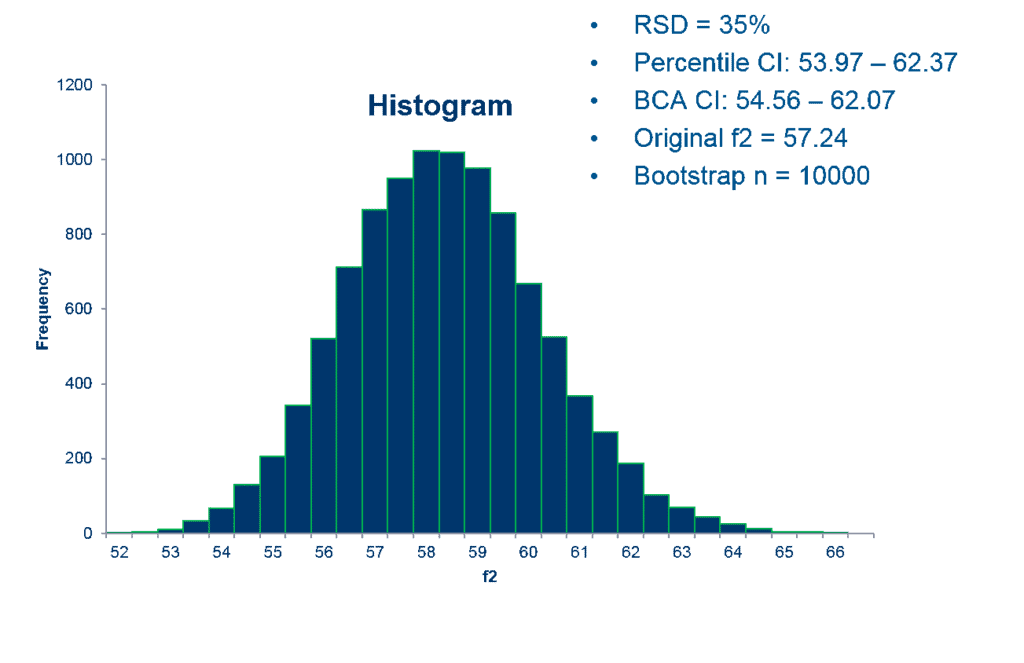
Is this method easy to implement?
For a statistician familiar with the SAS program and formulation sciences [or biopharmaceutics] in drug development, it may be. When the audience is familiar with highly variable dissolution data sets and is looking for an FDA-approved method, the bootstrap method with BCA is one.
You mention the FDA chooses to use the bootstrap method with BCA in the review process. Can you explain that?
We’ve learned the FDA has used the bootstrap method with BCA to reevaluate an applicant’s highly variable dissolution data set which did not demonstrate similarity (f2 < 50). After reevaluating the data set, the applicant passed based on the FDA’s recalculation using the bootstrap method with BCA. The applicant in that case was very lucky because they weren’t asked to reevaluate the dissolution dataset and resubmit the statistical method for dissolution data.
In the drug development process, the developer needs a very good method in place to make required comparisons, as the tests are being conducted to support drug development decisions and product approval, which ultimately can determine the viability and success of the of the product and its likelihood of reaching the market and the patients it is intended to treat.
Our expertise and experience with the statistical application of the bootstrap method with BCA in industry and with the FDA:
1) Scientific roundtable at AAPS, 2013
2) AAPS journal articles, 2015
3) Presentation at AAPS on bootstrap with BCA, 2015
To learn more about this topic, mark your calendars to attend a session at AAPS 2016, where Dr. Ruth Stevens and reviewers from the FDA will explore this topic further:
Dialogue and Debates Session: (#200) “Statistical Tests Applied To The Comparison Of Highly Variable Dissolution Profiles With Focus On Multivariate Statistical Distance (Msd) Vs. Bootstrap F2 Methods And Their Regulatory Application” for the 2016 AAPS Annual Meeting and Exposition. This session will be held on Wednesday, November 16, 2016 from 01:40 pm – 03:40 pm.
Using the Premier Consulting-preferred statistical bootstrapping method with BCA, we can help our clients get their statistics straight and their products approved. Learn more about the turnkey drug development services Premier Consulting can provide by contacting us today.
Author:
Ruth Stevens, PhD, MBA
Strategic Advisor